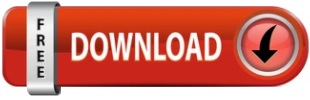
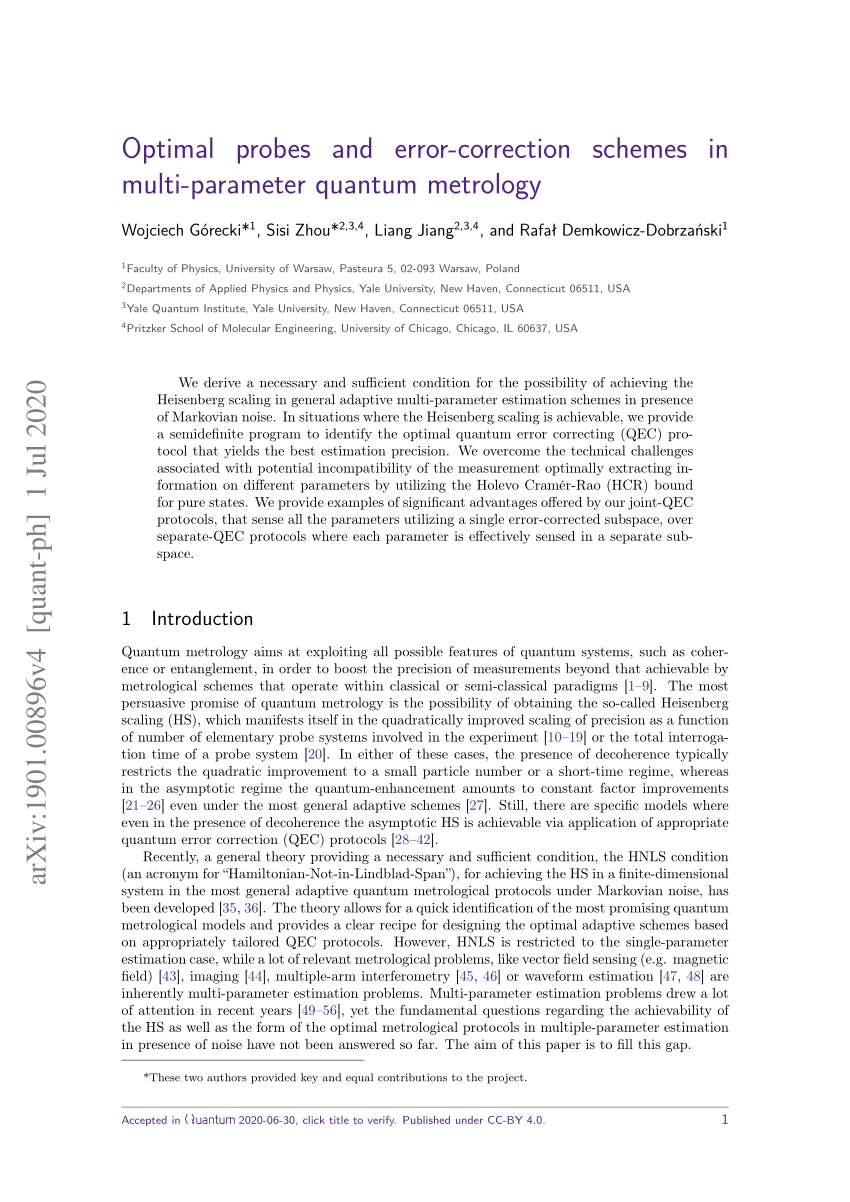
Finally, I provide fundamental bounds for multi-cycle recovery in the form of recurrence inequalities. I provide information-theoretic characterizations of the incomplete knowledge of the noise parameter to the lower bound, using both diamond distance and quantum Fisher information. The lower bound for the diamond distance has a simple form, and a potentially broader range of applicability in quantum information. Using generalized distinguishability measures as a starting point, I show that there is a fundamental bound in the performance of any recovery operation, as a function of the entanglement fidelity of the overall dynamics. In this article, I present information-theoretic bounds on the performance of such a spectator-based recovery. The memory qubits and the spectator system hence comprise the building blocks for a real-time (i.e. This necessitates the use of a "spectator" system, which makes an estimate of the noise parameter in real time, then feeds the outcome back to the recovery protocol as a classical side-information. However, such parameters are likely to drift in time in the context of implementing long-time quantum memories. Many recovery techniques, such as quantum error correction (QEC), rely on the apriori knowledge of the environment noise parameter to achieve their best performance. The recovery of fragile quantum states from decoherence is the basis of building a quantum memory, with applications ranging from quantum communications to quantum computing. Here, the authors report rigorous bounds on the generalisation error in variational QML, confirming how known implementable models generalize well from an efficient amount of training data. The power of quantum machine learning algorithms based on parametrised quantum circuits are still not fully understood. Our work injects new hope into the field of QML, as good generalization is guaranteed from few training data. Other potential applications include learning quantum error correcting codes or quantum dynamical simulation. We also show that classification of quantum states across a phase transition with a quantum convolutional neural network requires only a very small training data set. Our results imply that the compiling of unitaries into a polynomial number of native gates, a crucial application for the quantum computing industry that typically uses exponential-size training data, can be sped up significantly. When only K ≪ T gates have undergone substantial change in the optimization process, we prove that the generalization error improves to K/N. We show that the generalization error of a quantum machine learning model with T trainable gates scales at worst as T/N. In this work, we provide a comprehensive study of generalization performance in QML after training on a limited number N of training data points. Modern quantum machine learning (QML) methods involve variationally optimizing a parameterized quantum circuit on a training data set, and subsequently making predictions on a testing data set (i.e., generalizing).
#OPTIMAL RECOVERY QUANTUM ERROR CORRECTION CODE#
(c) Fault-tolerance: Finally, we address the question of whether such noise-adapted QEC protocols can be made fault-tolerant starting with a ] code and obtain a rigorous lower bound on threshold. (b) Application: As a simple application of noise-adapted codes, we propose an adaptive QEC protocol that allows transmission of quantum information from one site to the other over a 1-d spin chain with high fidelity. (a) Construction: Given a noise model, we propose a simple and fast numerical optimization algorithm to search for good quantum codes. In this spirit, we address the following questions concerning such adaptive quantum codes in this thesis. It is already known in the literature that such codes are resource efficient and perform on par with the standard codes. However, in cases where the noise structure in the system is already known, it might be more useful to consider quantum codes that are adapted to specific noise models. While it is very well known that quantum error correction (QEC) provides a way to protect against errors that arise due to the noise affecting the system, a perfect quantum code requires atleast five physical qubits to observe a noticeable improvement over the no-QEC scenario. A major obstacle towards realizing a practical quantum computer is the noise that arises due to system-environment interactions.
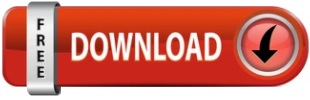